It takes about two hours to skim read all student comments of a single popular module of the Open University. But, reading alone is not enough to make sense of all those comments, which is a process that takes much longer. Everyone who has ever done a content analysis knows how much effort it takes to analyse texts. The OU has effective mechanisms in place to to act upon student comments on module level, but what about if we want to analyze larger aggregates, such as on qualification level or even faculty level for quality enhancement purposes? The large-scale analysis of student comments quickly becomes an intractable problem .
The Open University’s Text Analytics of Student Comments Initiative seeks solution to this problem and in my role as academic lead on this project I have been recently invited to speak at the QAA Scotland Enhancement Themes event: Improving the Student Experience, which was on the 30/04/2019 in Glasgow. The event brought together more than 100 delegates from mostly Scottish Universities, the industry, the QAA, AdvanceHE, and student associations. Clare Parks and her team did a tremendous job to prepare for this event. To give you a sense of the event, they gathered images and Tweets from the day: Wakelet on ‘Exploring Student Surveys event’.
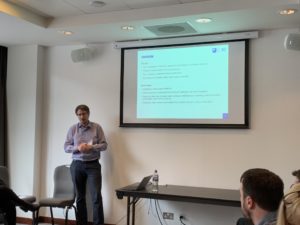
For my presentation, I had time to highlight results from two pieces of work out of a larger body of work that is already in the making since a few years.
Recently, I have been collaborating with the Open Programme team, which offers the OU Open Degree qualification. The Open Degree is a fantastic concept. A student can basically design their own qualification. It is all about openness extending the range of possibilities for our students. This programme fits well with our students, which can be seen by the number of students taking it. It is our biggest qualification.
Being the biggest qualification means also tons of student comments, which makes it a perfect example for the types of scenarios we want to explore within the Text Analytics of Student Comments Initiative. Based on technology that I have been developing, we looked at differences between students that studied towards an Open Degree and students that studied towards another named degree. The results of the analysis confirmed that Open Degree students emphasize in their comments certain topics more often than the rest of the students. They are, for example, very much interested in research, which seems very plausible for students that design their studies around their interests. They also mention more often their readings and course materials and they are very positive about those. The other students are also interested in these topics, it is just that Open Degree students mention these topics significantly more often. I touched on other topics, which I will not outline in detail here, because you can read about it in our latest Data Wrangler report .
The second example was about my collaboration with the Securing Greater Accessibility Initiative of the Open University. The OU has more students with disability than the entire student body of many Universities (currently about 25,000 students). The analysis showed that students that declared a disability talk about many student experience related things, but also that they talk about disability specific topics such as our DAISY books, screen reader technology, or comb binding books. Details of this study can be found in our contribution to the International Web for All conference, which also was a best paper candidate .
You can download the slides for my presentation here: https://www.enhancementthemes.ac.uk/en/current-enhancement-theme/student-engagement-and-demographics/national-student-survey-(nss)-analysis. It has many pointers to the reports and publications that I produced from this line of my research.
A big shout-out to my colleagues Heather Gibson and Shona Littlejohn from the Open University in Scotland, who made all of this possible. It is a fantastic feeling being part of this four nation OU family. Heather spotted this fantastic opportunity to further the discourse from our previous presentation at the QAA event in Edinburgh that looked more at the underlying technology.